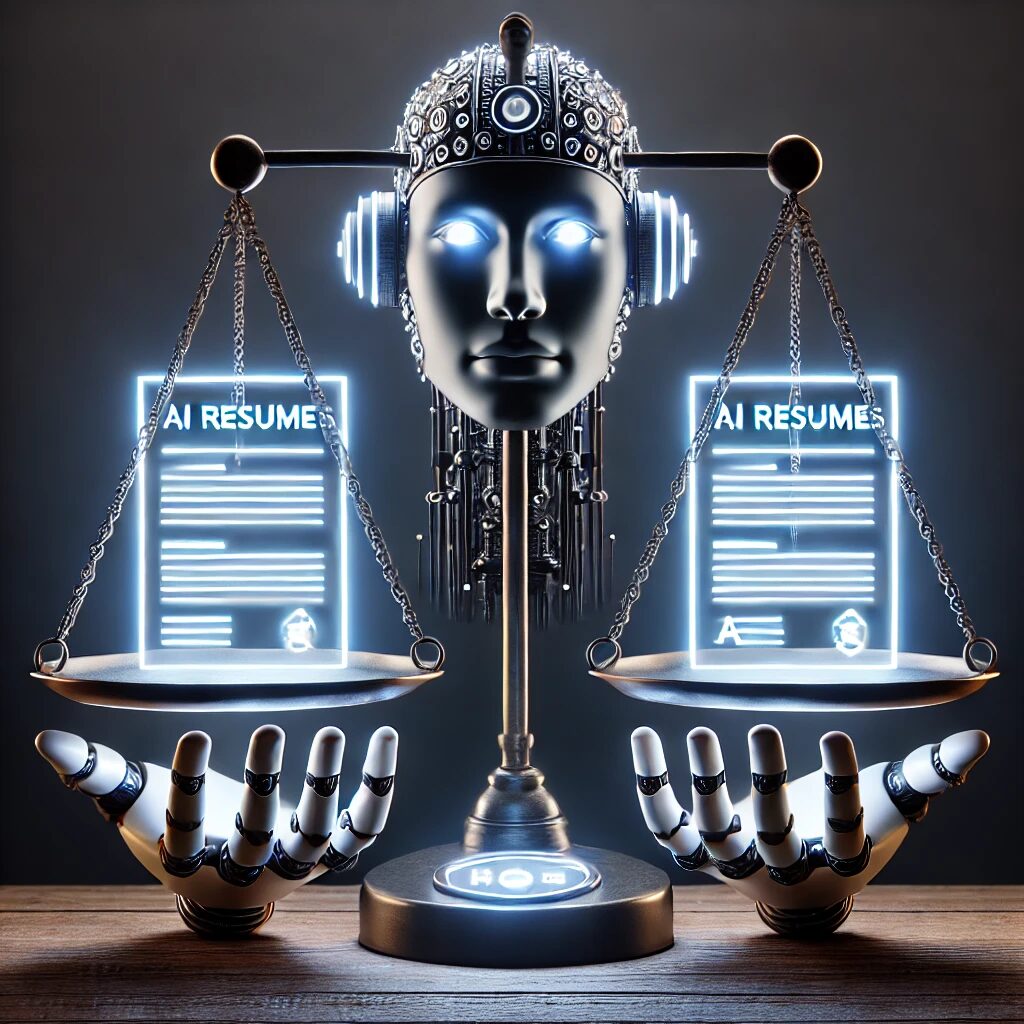
AI in Recruitment: Efficiency or Ethical Dilemma?
Imagine the time and energy companies can save by using AI-powered recruitment tools. It sounds promising, right? With the click of a button, resumes are scanned, shortlists are generated, and patterns are identified faster than a human ever could. AI cuts down on hiring costs, speeds up decisions, and potentially opens the door to a more efficient future in recruitment. But, there’s a twist.
While AI can scan a thousand resumes in seconds, can it make truly fair decisions? When we rely on technology for hiring, we often assume it’s objective and free from human error. But AI’s “objectivity” is only as good as the data it learns from. And herein lies the rub—if AI is trained on biased data, it ends up perpetuating those biases, whether we like it or not.
How Algorithms are Shaping the Hiring Process
AI is now a key player in recruitment. From resume parsing software to interview analytics, algorithms are everywhere. They spot keywords in resumes, assess facial expressions during interviews, and even analyze word choices in cover letters. These tools aim to help recruiters quickly and accurately determine who’s a good fit.
But while this seems like an efficient shortcut, it raises an important question: are we letting machines decide too much? AI doesn’t “know” your values or understand cultural nuances unless it’s taught to, which can lead to skewed results. Automated hiring systems risk amplifying biases if they weigh certain credentials, experiences, or backgrounds more heavily than others—often without us even knowing it.
The Promise of Fairness: Can AI Truly Be Unbiased?
AI promises something that humans struggle with: true objectivity. Since the 1960s, there’s been a desire to create fair hiring systems, free from prejudice based on race, gender, age, or background. In theory, AI can help achieve that. It evaluates candidates purely based on skills, experience, and qualifications.
However, AI models are built on historical data, and if that data contains bias, the AI will learn from it. If past hiring practices favored certain demographics over others, the algorithm might carry that over, unintentionally favoring the same profiles again. So, can AI actually be unbiased if it’s trained on biased human decisions?
The Hidden Dangers of Automating Human Judgment
By now, it’s becoming clear that handing over hiring decisions to an algorithm is not without its pitfalls. Even the smartest AI can miss key human elements—like potential or adaptability—qualities that don’t always show up in a resume. Human judgment is nuanced; it involves intuition, empathy, and context. Can we expect AI to replicate that?
Moreover, AI systems are often opaque. We might not fully understand how they make decisions. This lack of transparency becomes dangerous when bias creeps in, as it’s difficult to pinpoint where the problem lies. Imagine an AI favoring certain educational institutions or discarding applicants based on phrasing that appears too casual. Sounds unsettling, right?
Data Quality Matters: Feeding AI with the Right Inputs
A critical factor in automating hiring decisions is data quality. AI relies on massive amounts of historical data to make its assessments. The better the data, the more accurate the outcomes. But what happens if the data is flawed from the start?
If a company’s previous hiring records reflect biased decisions—consciously or not—those biases will seep into the AI model. For example, if more men than women were previously hired for tech roles, the AI may “learn” to associate men with better tech skills, even when women have equal qualifications.
This reinforces the age-old principle: garbage in, garbage out. If we want AI to be truly fair, we must feed it with diverse, balanced, and unbiased data. This means companies must actively review and correct past records, or else risk perpetuating old prejudices in shiny new tech.
Bias in, Bias Out: How Historical Data Skews AI
We’ve all heard the saying “history repeats itself,” and nowhere is this more evident than in AI hiring algorithms. If a company’s hiring patterns have historically leaned towards a certain demographic, the AI will follow suit. The technology isn’t inherently biased, but it learns from the past. And if the past was skewed, so too will the future be.
For instance, imagine a scenario where a financial firm has traditionally hired more graduates from Ivy League schools. If that pattern is reflected in their data, the AI might assume that Ivy League grads are the best candidates, favoring them disproportionately. This bias in hiring becomes automated, even though it may not align with the company’s current goals of increasing diversity or inclusivity. The AI is not smart enough to know that patterns shouldn’t always be replicated—it just learns them.
The danger of this is clear: AI amplifies and scales biases at a faster rate than any human could. This is why, when developing or implementing AI for hiring, companies need to keep a close eye on the data they input. If the data is skewed, the outcomes will be too.
Transparency in AI: Understanding the Black Box
One of the most frustrating aspects of AI is that it often functions like a black box. You input data, and it spits out a decision, but you don’t always know why or how it arrived at that conclusion. This lack of transparency is especially problematic when AI is used in hiring. Candidates may be rejected without understanding the reason, and recruiters may not be able to fully explain how the AI made its choices.
The problem here is twofold: first, AI lacks the ability to articulate its decisions. Second, many companies do not fully understand the models they are using. This opacity creates an environment where biased decisions could go undetected for long periods. For example, a hiring algorithm might consistently reject women for technical roles based on subtle cues in resumes that the AI learned to associate with “weaker” candidates. Without transparency, it’s almost impossible to challenge or correct this bias.
To mitigate this, companies must demand explainability from their AI providers. AI systems should be able to provide reasoning behind their decisions, allowing companies to evaluate whether they are making fair choices.
AI and Diversity: A Double-Edged Sword?
AI has been hailed as a tool for fostering workplace diversity, but is it truly a silver bullet? While algorithms can certainly help reduce blatant discrimination, they can also subtly reinforce existing biases. In fact, some recruitment tools, though marketed as promoting diversity, end up doing the opposite.
For instance, some AI systems may prioritize certain words or phrasing in resumes, unintentionally favoring specific demographics. If a job description includes terms more commonly used by men, the AI might shortlist male candidates more frequently. Similarly, algorithms could downplay resumes from candidates with non-traditional educational backgrounds, assuming that they don’t match the “ideal” profile—again, based on historical patterns.
It’s important to remember that AI can’t inherently champion diversity. That responsibility lies with the people who design, train, and monitor these systems. Companies need to regularly audit AI outputs and make adjustments to promote genuine inclusivity, or they risk reinforcing the very biases they aim to eliminate.
Human Oversight: The Key to Ethical AI in Hiring
Even the best AI systems need a human touch. While AI can handle the heavy lifting of processing large volumes of data, it should never completely replace human judgment. There are nuances in the hiring process—such as cultural fit, motivation, and potential—that an algorithm can’t fully understand.
By integrating human oversight into AI hiring decisions, companies can catch and correct errors that the algorithm may overlook. Recruiters should review AI-generated shortlists, ask critical questions about the fairness of the selections, and provide feedback to improve the system. This checks-and-balances approach ensures that AI remains a tool, rather than the sole decision-maker.
Think of AI as a helpful assistant, not the boss. Let the machine do the math, but let the people make the final call.
Can AI Replace Recruiters? The Human Touch in Decision-Making
The rise of AI in hiring naturally brings up the question: will AI replace human recruiters? The answer, at least for now, is no. While AI can streamline processes and provide valuable insights, it can’t replace the unique abilities of human recruiters.
AI is great at identifying patterns and matching keywords, but it can’t understand a candidate’s passion or growth potential. It doesn’t consider a candidate’s ability to adapt or learn new skills, qualities that might be crucial for certain roles. The empathy and intuition humans bring to the hiring process are invaluable. After all, hiring isn’t just about checking boxes—it’s about finding the right fit for a company’s culture and values.
Recruiters can also build relationships with candidates, offering reassurance, answering questions, and providing feedback—things AI can’t do. So while AI may assist in sifting through resumes or scheduling interviews, the human touch will remain essential in making final decisions.
Legal Ramifications: Who’s Responsible When AI Gets it Wrong?
When AI makes a biased hiring decision, the blame can get tricky. If a company unknowingly uses an AI tool that discriminates based on race, gender, or another protected class, who’s liable? Is it the tech provider that built the system or the company using it? The legal landscape is still catching up to the rapid development of AI, and the question of accountability is far from settled.
In many cases, companies using AI systems may be held responsible for the actions of those systems. Discriminatory hiring practices, whether caused by human prejudice or machine learning bias, could expose a business to lawsuits and reputational damage. The legal standards governing AI in hiring are evolving, but companies are expected to ensure that their tools comply with equal employment laws.
This is why due diligence is critical. Employers need to be aware of how their AI systems work, consistently audit them for fairness, and ensure transparency. If a candidate files a lawsuit claiming discrimination due to an AI-based decision, the company must be prepared to prove that the system was fair and compliant with regulations.
Companies Leading the Way in Ethical AI
While AI bias is a genuine concern, some forward-thinking companies are already taking steps to ensure their AI systems are used ethically. Google and IBM have led the charge, creating tools and frameworks for mitigating bias in AI systems. Google’s AI fairness tools allow businesses to evaluate their algorithms for unintended biases, while IBM has introduced AI OpenScale, which monitors AI models for fairness and provides explainability in decision-making.
Other companies are going even further by incorporating diversity-focused features into their AI recruitment systems. For example, Pymetrics, a recruitment platform, uses neuroscience-based assessments to predict candidates’ success based on cognitive and emotional abilities—without considering gender, race, or background. Pymetrics regularly tests their models to ensure fairness and diversity in outcomes.
These pioneers in ethical AI are proving that it’s possible to harness the power of algorithms while reducing bias. However, ethical AI isn’t something that can be built once and forgotten; it requires ongoing vigilance, testing, and adjustments as technology and workplaces evolve.
Ethical AI: Best Practices for Businesses
Creating and implementing ethical AI in hiring takes more than good intentions. There are a few key practices that companies can follow to ensure their AI tools promote fairness rather than perpetuate bias:
- Diverse data sources: Use training data that reflects the diversity you want in your workforce. This reduces the risk of algorithms learning and repeating historical biases.
- Regular audits: Continuously monitor AI outputs for signs of bias. Evaluate how the system treats candidates from different demographics and make adjustments as needed.
- Explainability: Choose AI systems that offer transparency. Recruiters should be able to explain how decisions are made and why certain candidates were selected or rejected.
- Human oversight: As mentioned earlier, AI should never be a standalone decision-maker. Pair algorithmic outputs with human judgment to ensure fairness in hiring.
- Clear accountability: Establish clear lines of responsibility in case of discrimination claims. Companies must know who is accountable if the AI system makes a biased decision—whether it’s the vendor or the internal team.
By implementing these best practices, businesses can build AI systems that support diversity and fairness in hiring, while still benefiting from the efficiency and speed these tools provide.
The Future of AI in Hiring: Finding the Right Balance
The future of AI in hiring will likely depend on our ability to strike a balance between automation and human oversight. As AI continues to evolve, its role in recruitment will become more sophisticated. We might see systems that can better assess soft skills, cultural fit, and adaptability, which are areas where AI currently struggles. But even as AI advances, it will never be perfect.
The key will be ensuring that AI systems are constantly updated to reflect evolving ethical standards. Regulations will also play a significant role, with governments likely stepping in to ensure that AI-driven hiring systems comply with anti-discrimination laws. As companies adopt AI, they must be proactive in addressing potential biases and ensuring transparency and fairness.
It’s safe to say that AI won’t replace humans in the hiring process any time soon. Instead, the future of AI in recruitment will involve a collaborative approach, where algorithms assist humans in making better, more informed decisions.
Can AI Create a More Diverse Workforce?
The big question is: can AI actually help build a more diverse workforce? The answer isn’t simple. On the one hand, AI has the potential to eliminate some of the unconscious biases that humans bring to the table. If programmed and trained correctly, algorithms can evaluate candidates based purely on qualifications and potential, rather than gender, race, or other irrelevant factors.
On the other hand, AI is only as good as the data and training it receives. If a system is built on biased data or lacks diversity in its models, it could end up perpetuating the very biases it’s supposed to eliminate.
To truly create a more inclusive workforce, companies must take an active role in shaping how their AI systems operate. This involves regular testing, human oversight, and a commitment to ethical AI practices. When used responsibly, AI can help identify talent from unexpected places, opening doors to a more diverse range of candidates.
Conclusion: Striking a Balance Between Efficiency and Fairness
In the end, AI in hiring is both a blessing and a challenge. It has the potential to make recruitment faster, more efficient, and less prone to human error. But it also runs the risk of automating the very biases it’s supposed to eliminate. The future lies in finding a balance—leveraging the strengths of AI while keeping human judgment at the forefront. Companies that invest in transparent, ethical AI systems will not only improve their hiring processes but also build a more diverse and inclusive workforce. And that’s a win for everyone.
Resources
Harvard Business Review: “How to Reduce Bias in AI Hiring”
Website: hbr.org
This article provides insights into the challenges and potential solutions for reducing bias in AI-driven recruitment tools.
Equal Employment Opportunity Commission (EEOC)
Website: www.eeoc.gov
The EEOC offers guidelines and legal standards regarding discrimination in hiring practices, which companies using AI must comply with.