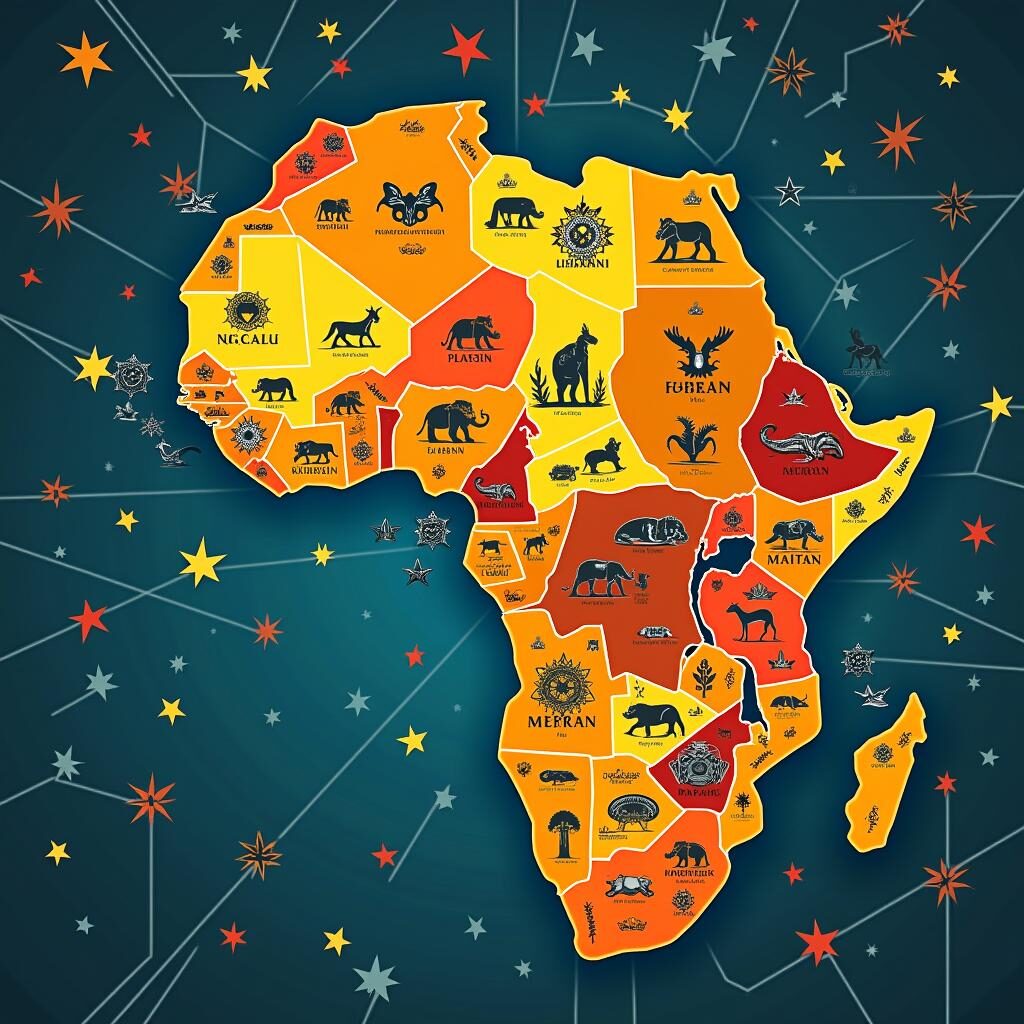
Many African languages remain underrepresented in AI. Despite being home to over 2,000 languages, Africa’s linguistic diversity has historically been excluded from the digital landscape. Fortunately, this trend is changing.
Here’s how NLP is bridging the gap for African languages and what it means for the future.
The Importance of African Languages in Technology
Preserving Cultural Identity Through Language
Language is the soul of culture. African languages like Yoruba, Swahili, and Zulu embody the histories, stories, and traditions of millions.
Ignoring these languages in AI perpetuates digital inequality and cultural erasure. NLP’s potential to preserve cultural identity offers hope for reversing this trend.
By enabling tech to understand and process African languages, we can digitize and archive endangered dialects. This ensures their survival for future generations.
Bridging the Digital Divide
The global tech revolution has excluded non-dominant languages, creating a digital divide.
Imagine a farmer in rural Tanzania who could access agricultural information in Swahili, or a student in Senegal learning in Wolof online.
NLP makes tech accessible to people who don’t speak global lingua francas like English or French. It’s a game-changer for economic development and education.
Strengthening Local Economies
AI applications tailored to African languages can unlock new markets and innovations. E-commerce platforms, for example, could thrive by serving users in local languages, empowering small businesses and entrepreneurs.
Challenges in African Language NLP
Limited Data Availability
African languages often lack the large datasets needed for NLP training.
Unlike English, for which there are billions of online documents, African languages may have sparse digitized text.
To address this, organizations are turning to community-driven data collection efforts, such as crowdsourced translations and corpora.
Linguistic Complexity
Many African languages feature tones, complex verb systems, and regional variations.
For instance, Bantu languages like Xhosa use click consonants, which challenge traditional NLP models.
Creating algorithms to accurately process these unique structures requires innovative linguistic engineering and contextual understanding.
Resource Constraints
AI research often concentrates in wealthier nations, leaving African languages behind. Limited funding, infrastructure, and expertise in AI fields exacerbate this gap.
Collaborations between tech companies, governments, and academic institutions are essential for progress.
Current Innovations in NLP for African Languages
Projects and Partnerships
Organizations like Masakhane and AI4D Africa are leading the charge. Masakhane, a grassroots initiative, is building open-source NLP tools for African languages, from translation models to sentiment analysis tools.
These projects demonstrate the power of open collaboration, enabling researchers from across the globe to contribute to African NLP.
Leveraging Transfer Learning
Transfer learning allows AI systems trained in dominant languages to be adapted for underrepresented ones.
For example, using models like BERT or GPT as a foundation, researchers can tweak these frameworks for Swahili, Hausa, or Amharic with fewer data requirements.
Community-Led Data Collection
Communities are stepping up to create datasets. Efforts include:
- Translating open-source texts.
- Documenting oral histories.
- Building annotated language corpora.
These grassroots contributions are fueling breakthroughs in NLP and helping AI become more inclusive.
The Role of AI in Empowering African Communities
Enhancing Education Accessibility
With NLP-driven tools, language barriers in education can crumble.
AI-powered translators and voice assistants are making it easier for learners to access educational materials in their native languages. Imagine online courses offered in Xhosa, Somali, or Igbo, reaching millions who previously lacked this opportunity.
Voice recognition systems tailored for African accents and dialects are also transforming literacy programs, helping people learn to read and write in their local languages.
Boosting Healthcare Outcomes
In many rural areas, access to healthcare depends on effective communication. NLP tools can improve the experience by translating medical instructions or symptoms into a patient’s native language.
For example, health chatbots could assist Swahili-speaking patients in understanding prescriptions or provide Hausa speakers with preventative health tips.
AI advancements are saving lives by improving patient-doctor interactions, especially in underserved regions.
Facilitating Governance and Civic Engagement
Language inclusivity in governance promotes transparency and citizen participation. Imagine accessing government documents, laws, or voting materials in Zulu or Twi, not just English or French.
AI is empowering citizens by enabling NLP-based tools for legal translations and public information dissemination in multiple languages.
Future Prospects for African Languages in AI
Building Multilingual AI Assistants
Tech giants are starting to focus on multilingual AI that supports underrepresented languages. Tools like Google Translate and Microsoft Translator have expanded to include Swahili, Yoruba, and others.
But the future lies in conversational agents fully capable of interacting in African languages, considering idioms, proverbs, and cultural nuances.
AI and Content Creation
NLP models can aid African writers and creators in producing content in native languages. Whether generating subtitles for Nollywood films or creating e-books in Amharic, AI empowers cultural content to flourish.
This also paves the way for a richer online presence of African languages, balancing the digital landscape.
A Rising Demand for Ethical AI
As African language NLP evolves, ethical questions will emerge.
- Who owns the datasets used to train AI?
- How are languages represented without bias?
Stakeholders must prioritize fairness and transparency while uplifting African voices.
AI In Africa: Turning Challenges Into Opportunities
Collaborations Paving the Way
Tech Companies Partnering with Local Researchers
Companies like Google, Meta, and DeepMind are funding African NLP research. These partnerships help combine global expertise with local insights, ensuring that solutions are both innovative and relevant.
For example, Google’s AI initiative for Africa is producing multilingual datasets for low-resource languages, while DeepMind’s collaborations with universities drive academic research.
NGOs and Grassroots Efforts
Organizations like LinguAfrica and Sankore AI work directly with communities, capturing spoken and written language data.
These efforts highlight the importance of local participation in AI’s development journey, fostering a sense of ownership and inclusivity.
Insights into Opportunities and Barriers
The Role of Language in Shaping Perception
Cultural Nuances Beyond Translation
Language isn’t just about communication; it shapes how communities think and interact. For instance, African languages often incorporate rich metaphorical and idiomatic expressions, which are deeply tied to their cultural contexts.
Take the Yoruba phrase, “Ọmọ ilẹ́kùn ríru kò gbọ́do ṣe aṣálẹ̀ fún ojú ènìyàn,” roughly translating to “A person known for opening doors must not betray trust.” Direct translations fail to capture the cultural wisdom embedded in these expressions.
For NLP to succeed, it must go beyond word-for-word translations to context-aware understanding, requiring models trained on cultural subtleties.
The Sapir-Whorf Perspective in AI
The Sapir-Whorf hypothesis, which suggests that language shapes worldview, underlines the need to include African languages in AI systems. Exclusion perpetuates a monocultural perspective, alienating entire communities from contributing to global narratives.
AI systems trained on African languages bring diverse cognitive frameworks to machine understanding, enriching global AI ecosystems.
Barriers in African Language NLP: A Closer Look
Structural and Orthographic Challenges
African languages feature unique grammatical structures that diverge from Western linguistic norms.
- Tonal Variations: Languages like Yoruba and Igbo rely on tone to convey meaning. A single word can mean entirely different things depending on pitch.
- Agglutination: Swahili and other Bantu languages rely heavily on prefixes, suffixes, and infixes, creating long compound words that defy conventional tokenization methods in NLP.
For example, in Swahili:
- Ninapenda means “I love.”
- Nitapenda means “I will love.”
Training models to account for these morphological changes requires adaptive tokenization algorithms.
Dialects and Regional Variations
Africa’s linguistic diversity isn’t just about the number of languages; it’s also about the complexity within them. Dialects and regional accents further complicate the NLP landscape.
For instance:
- Arabic in Africa: Variants like Darija (Moroccan Arabic) differ significantly from Classical Arabic in grammar, syntax, and vocabulary.
- Hausa and Twi: Different regions emphasize unique pronunciations and vocabulary, requiring tailored training data for NLP models to ensure comprehensive coverage.
This diversity demands models that are flexible enough to understand variations while maintaining consistent accuracy.
Current NLP Models: Strengths and Limitations
The Use of Multilingual Pretrained Models
Multilingual NLP models, like mBERT (Multilingual BERT) and XLM-R (Cross-lingual Roberta), are gaining traction in African language processing. These models are trained on data spanning multiple languages, offering a good starting point for underrepresented ones.
Strengths
- Transfer Learning: Pretrained models designed for French or Arabic can adapt to related African languages, leveraging overlapping linguistic features.
- Reduced Training Costs: With minimal data, these models can achieve reasonable results for low-resource languages like Luganda or Tigrinya.
Limitations
- Bias Propagation: These models often carry biases from dominant languages, which can skew translations or sentiment analysis for African contexts.
- Data Gaps: Without adequate local data, even the best models fail to represent nuances, leaving gaps in linguistic richness.
The Push for Monolingual Models
There’s growing advocacy for monolingual NLP models trained solely on African languages. Such models can:
- Handle linguistic specificity better than general multilingual models.
- Capture cultural expressions and idiomatic phrases that are unique to a single language.
Grassroots Innovations in African NLP
Harnessing Oral Histories
In Africa, oral traditions dominate, offering a wealth of untapped linguistic data. Efforts to transcribe and digitize oral histories are invaluable for NLP training.
Examples in Action
- Voice Data Platforms: Projects like Mozilla’s Common Voice have crowdsourced audio clips from speakers of languages like Luganda and Wolof, creating datasets for speech-to-text systems.
- Storytelling Archives: Digital storytelling platforms are turning local folklore into annotated datasets, preserving narratives while training NLP models.
These initiatives are not just about creating functional datasets—they’re about preserving the essence of African identity.
AI-Driven Linguistic Research
AI is aiding linguists in studying undocumented languages. Through unsupervised machine learning, researchers can analyze linguistic patterns in under-documented dialects, creating preliminary grammars and dictionaries.
For example:
- Algorithms analyzing phonetic patterns can predict likely grammatical structures, enabling rapid language mapping.
Economic and Social Impacts
Unlocking New Market Potential
Localized NLP can catalyze market growth by reaching underserved communities.
- E-commerce in Local Languages: Platforms targeting native speakers can improve user engagement and conversion rates.
- Local Advertising: NLP can enable better contextual targeting for ads, ensuring culturally relevant marketing strategies.
Case Study: Mobile Payments
Services like M-Pesa succeeded partly by offering interfaces in Swahili, demonstrating how language inclusivity drives adoption. Extending such services to include voice and text interfaces in other African languages could revolutionize access to digital finance.
Empowering Women and Marginalized Groups
In many African societies, language plays a key role in gender dynamics. NLP tools that prioritize local languages can:
- Empower women in rural areas by offering digital tools for healthcare, education, and small business management.
- Break barriers for marginalized communities by enabling them to interact with government and social services in their native tongues.
Ethical Considerations
Data Ownership and Privacy
African NLP projects often rely on community-generated data. However, who owns this data becomes a crucial question.
- Fair Compensation: Communities must benefit from the commercial use of their language data.
- Open Access: Public datasets should remain accessible to prevent monopolization by corporations.
Representation in AI Development
Linguistic communities must be involved in creating the tools that represent them. Without their input, there’s a risk of perpetuating misrepresentation and exclusion.
Conclusion: A New Chapter for African Languages
The marriage of AI and African languages is breaking barriers, empowering communities, and preserving heritage.
As NLP continues to evolve, the voices of Africa’s people will echo louder in the digital world, enriching global innovation and cultural diversity.
FAQs
What are the most promising NLP tools for African languages?
Promising tools include:
- Google’s BERT models: Used for tasks like translation and text classification in Swahili.
- Microsoft Translator: Supports languages like Amharic and Hausa for real-time translations.
- Masakhane Models: Open-source resources for text summarization, sentiment analysis, and machine translation.
These tools are evolving, but their success depends on continued data collection and community support.
Can NLP help preserve endangered African languages?
Absolutely! By digitizing and archiving endangered languages, NLP ensures their survival. For example:
- Transcribing oral histories in languages like Khoekhoe creates text datasets for educational use.
- AI algorithms trained on indigenous languages like Tsonga help researchers study their grammar and structure.
Such initiatives safeguard cultural heritage while empowering younger generations to reconnect with their roots.
What role do communities play in African NLP development?
Communities are the backbone of African NLP. They contribute by:
- Recording spoken data: Speakers of Igbo or Twi provide audio clips to build speech recognition systems.
- Translating texts: Volunteers translate open-source materials into local languages for training datasets.
For example, Mozilla’s Common Voice project relies on native speakers to create audio corpora for lesser-known languages like Tigrinya.
This collaborative effort ensures that language technology serves the people who need it most.
How does NLP address African dialects and regional variations?
NLP tackles dialects and regional variations by creating models trained on diverse datasets from multiple regions.
For instance:
- Arabic Variants: Darija (North African Arabic) differs significantly from Egyptian Arabic. NLP systems are being fine-tuned for these distinctions using region-specific corpora.
- Bantu Languages: Swahili NLP tools incorporate datasets from Kenya, Tanzania, and Uganda to capture regional lexical differences.
To make systems more inclusive, researchers use adaptive learning algorithms that handle variations within a language.
Are there economic benefits to investing in African NLP?
Yes, the economic benefits are enormous. Localized NLP can unlock markets by enabling native-language services:
- Retail and E-commerce: NLP-driven chatbots in languages like Yoruba or Amharic enhance customer interactions and improve sales.
- Financial Services: Voice recognition tools in Hausa help customers access banking services without needing English or French fluency.
These technologies promote inclusion and allow businesses to tap into vast, underserved markets across the continent.
How is AI being used to teach African languages?
AI tools like interactive apps and NLP-powered language tutors are transforming how African languages are taught. Examples include:
- Duolingo Swahili: Offers lessons tailored for beginners, leveraging AI for adaptive learning.
- KiswahiliKit: Uses NLP to generate grammar exercises and quizzes for Swahili learners.
These applications cater to both native speakers seeking literacy skills and foreigners interested in learning African languages.
What innovations are addressing the lack of data for African languages?
Several innovations are solving data scarcity:
- Crowdsourced Platforms: Projects like Masakhane and Common Voice gather text and audio directly from native speakers.
- Data Augmentation: AI generates synthetic data by altering existing text to create diverse examples, such as tone or dialect variations.
- Speech-to-Text Conversion: Recording oral histories in languages like Wolof creates datasets for text-based NLP applications.
These strategies are laying a strong foundation for NLP advancements in African languages.
How can governments support NLP development for African languages?
Governments can play a key role by funding projects, enacting policies, and fostering collaborations:
- Policy Support: Mandating multilingualism in AI systems for government services ensures accessibility.
- Funding Initiatives: Providing grants for linguists and developers to create NLP tools tailored to local needs.
- Public-Private Partnerships: Collaborating with tech companies to accelerate the development of African language technologies.
For example, the South African government has supported NLP projects for isiZulu, enabling wider use in public services and education.
Resources for Exploring African Languages in NLP
Open-Source Platforms and Tools
Masakhane
A grassroots initiative focused on building NLP tools for African languages. It provides open-source machine translation models and datasets for over 50 languages.
- Website: Masakhane
Common Voice by Mozilla
A global project collecting voice data, including contributions from African language speakers like Swahili, Wolof, and Luganda.
- Website: Mozilla Common Voice
African Storybook Project
This platform provides free access to children’s stories in African languages, creating valuable text corpora for NLP training.
- Website: African Storybook
NLP Frameworks and Models
Hugging Face Transformers
Hosts pre-trained multilingual models like mBERT and XLM-R, adaptable to African languages with fine-tuning.
- Website: Hugging Face
Google BERT Multilingual Models
Google offers multilingual BERT models that can be customized for African languages through transfer learning.
- Documentation: Google Research BERT
FastText by Facebook
A library for efficient text classification and word embedding, suitable for resource-scarce languages.
- Website: FastText
Research Papers and Publications
AfricaNLP Workshop Proceedings
Annual workshops featuring papers and presentations on advances in African NLP. Topics include translation, sentiment analysis, and text-to-speech technologies.
- Website: AfricaNLP Workshop
“Pretraining Multilingual Neural Machine Translation Models with African Languages”
This paper explores the use of multilingual models to address African NLP challenges.
- Read on arXiv
“The State and Challenges of African NLP”
A comprehensive survey highlighting the state of African NLP research and tools.
- Read on arXiv
Online Communities and Forums
AI4D Africa
A network supporting artificial intelligence research and applications for African development, including NLP initiatives.
- Website: AI4D Africa
Kaggle Datasets Community
Kaggle hosts several African language datasets, including corpora for Swahili, Hausa, and Yoruba, contributed by researchers worldwide.
- Website: Kaggle Datasets
Linguistics and African Languages on Reddit
Subreddits like r/linguistics or r/Africa discuss African language preservation, AI applications, and emerging NLP projects.
- Explore: Reddit Linguistics
Training and Learning Resources
Coursera: Natural Language Processing Specialization
Courses covering the fundamentals of NLP, including hands-on projects where students can apply skills to African languages.
- Website: Coursera NLP Specialization
Masakhane NLP Tutorials
Community-led tutorials on building translation models, text summarization tools, and sentiment analyzers for African languages.
- Learn: Masakhane Tutorials
Speech and Language Processing by Daniel Jurafsky and James H. Martin
A foundational textbook in NLP, offering insights into methods that can be adapted for African languages.
Details: Jurafsky-Martin NLP Textbook