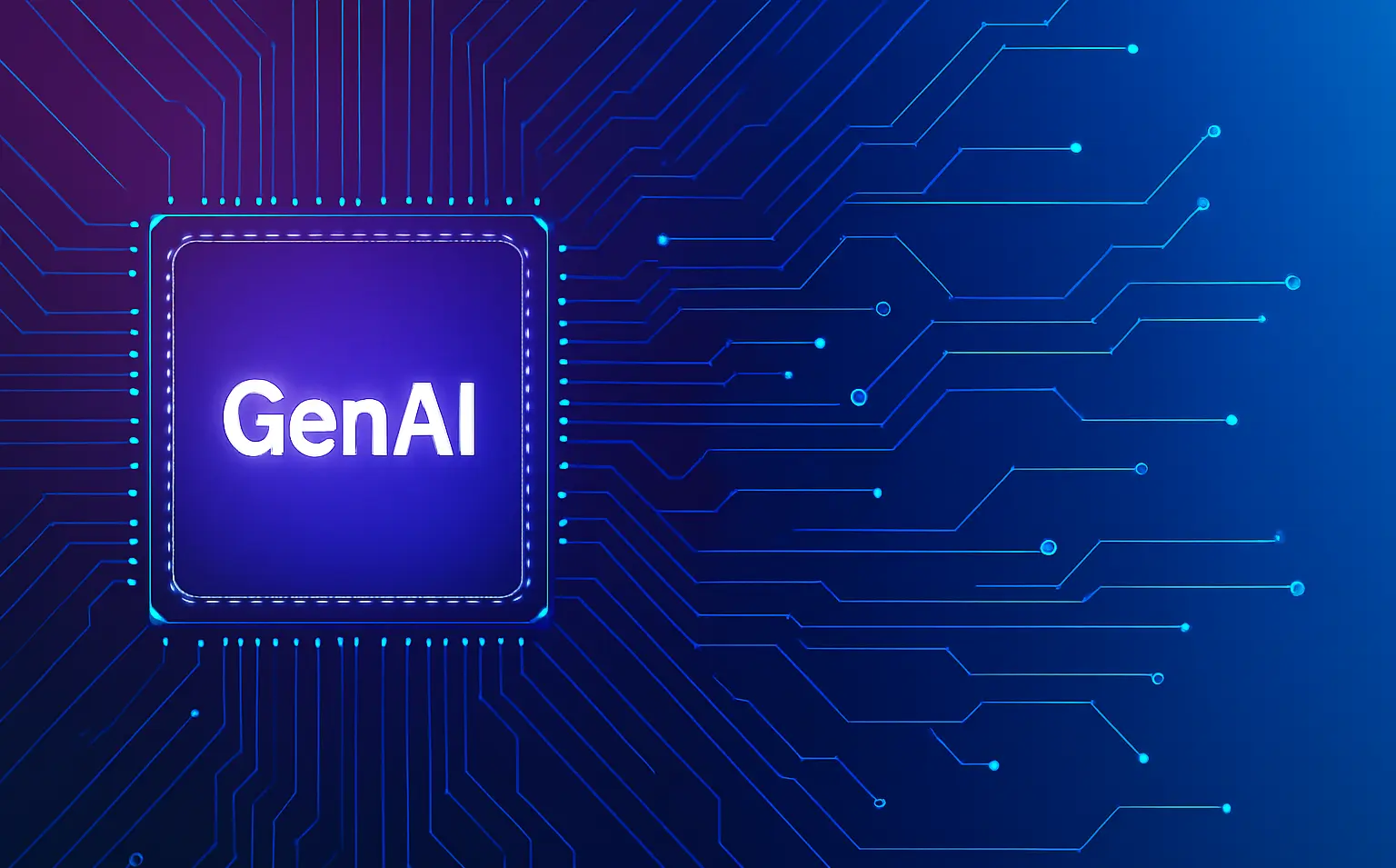
The Rise of Machine Hypothesis Generation
In labs across the globe, something fascinating is unfolding: machines aren’t just helping scientists—they’re starting to lead them. With advances in generative AI, we’re witnessing a shift where algorithms are proposing original scientific hypotheses.
This isn’t science fiction anymore. Platforms like IBM’s Watson and emerging AI research tools now suggest testable ideas by analyzing massive data sets. Instead of only supporting discovery, GenAI is inching toward initiating it.
By scanning thousands of academic papers, AI can detect hidden patterns even seasoned researchers miss. Then, it proposes questions worth investigating. That’s a serious game-changer.
How GenAI Is Trained to “Think” Like Scientists
Training an AI to formulate hypotheses isn’t like teaching it to play chess. It’s messier. Scientific thinking is complex, and data isn’t always neat or structured.
To cope, GenAI models are fine-tuned on vast scientific corpora—millions of journal articles, lab reports, and datasets. They don’t just mimic sentences; they learn the structure of reasoning. This enables them to infer plausible cause-effect relationships.
Some systems are even trained using reinforcement learning, rewarding accurate predictions or novel, testable ideas. That’s where they start looking less like tools and more like research partners.
The First AI-Proposed Discoveries Are Already Here
GenAI isn’t just throwing out random ideas—it’s already proven useful. In drug discovery, AI has identified molecular structures for rare diseases years ahead of human timelines.
In 2020, an AI developed by researchers at Stanford hypothesized a new antibiotic, later confirmed in lab trials. It did this by scanning chemical interactions faster than any human team could manage.
That success isn’t isolated. More labs are confirming AI-generated theories that would’ve taken decades with traditional research.
Shifting Roles: Scientists as Reviewers, Not Inventors?
This begs a provocative question—what if future scientists act more like curators or reviewers of machine-born ideas?
Instead of generating hypotheses from scratch, researchers might guide AI models, then choose which ideas are worth pursuing. That’s not a downgrade—it’s a redistribution of creative effort.
Rather than drowning in data, scientists can focus on what matters most: interpretation, validation, and application. But of course, this shift also raises philosophical and ethical dilemmas about authorship and credit.
Key Takeaways
- GenAI can now formulate testable hypotheses, not just analyze data.
- It’s trained on vast scientific literature to mimic structured reasoning.
- AI-generated ideas are already leading to real-world discoveries.
- The role of scientists may evolve into interpreters and curators of AI output.
Is Creativity Still Uniquely Human?
In the next section, we’ll explore a deeper question—can a machine truly be creative? Or is it just remixing what we’ve already discovered? Let’s peel back the layers of what “innovation” really means when algorithms are behind the curtain.
Can Machines Be Creative? Rethinking Innovation with GenAI
What Counts as Scientific Creativity?
We tend to romanticize creativity—especially in science. The image of a lone genius struck by a eureka moment still dominates our imagination. But creativity, at its core, is about recombining known elements into something useful or novel. That’s exactly what GenAI excels at.
AI doesn’t have intuition. It doesn’t dream. But it can simulate the appearance of creativity by analyzing trillions of data points and spotting unheard-of connections. Sometimes, that’s all you need to trigger a genuine breakthrough.
So maybe the real question isn’t “Is AI creative?”—it’s “Is human creativity as mystical as we think?”
Innovation Without Intent: Is That Still Innovation?
Let’s be real—machines don’t have intentions or goals. They’re not trying to solve cancer or change the world. They just process input and generate output based on probability and structure.
Yet, some of that output is sparking discoveries that change lives. In that sense, we might need to expand our definition of innovation to include idea generation without intent.
This shift feels uncomfortable. But it’s also freeing. Because it shows that progress doesn’t always need a human origin story—sometimes it just needs good algorithms.
The Human-AI Duo: Blending Intuition and Computation
Where GenAI shines in brute-force analysis, humans still dominate in judgment, intuition, and ethical reasoning. That’s why the most powerful discoveries emerge from collaboration, not competition.
Think of it like jazz. AI lays down the pattern. Humans riff off it. Together, they form a rhythm that neither could create alone.
This kind of hybrid creativity is already being used in climate science, biomedical engineering, and material design. We’re learning that the smartest research doesn’t always come from solo acts—but from duets.
Did You Know?
- DeepMind’s AlphaFold solved the protein folding problem—a biological mystery for 50 years—in just months using AI inference.
- Some AI models are generating scientific illustrations and experiment blueprints automatically, based on textual input.
- AI-driven labs, like the University of Liverpool’s “robot scientist,” can test hypotheses 24/7, adjusting experiments on the fly.
What Happens When AI Gets It Wrong?
Up next, we’ll tackle a more sobering angle—what are the risks when machines mislead us? From flawed models to bias in training data, let’s explore the dark side of AI-led discovery.
When AI Gets It Wrong: Risks of Machine-Led Discovery

The Danger of Flawed Data Foundations
AI’s brilliance is only as good as its input. And let’s face it—science is messy. Datasets can be biased, incomplete, or even outdated. If GenAI models learn from flawed or skewed data, their hypotheses may be dangerously misleading.
This has already happened. In fields like genetics and pharmacology, AI systems have proposed treatments later found to be toxic or ineffective. Not because the algorithm failed—but because its training materials lied to it.
When machines hallucinate patterns that aren’t real, scientists can chase phantoms for months—or worse, base clinical trials on shaky ground.
Ethics in the Age of AI Hypothesis Generation
As GenAI becomes a more active player in science, ethical questions flood in. Who owns an AI-generated discovery? Can a machine be listed as a co-author? Who’s liable if it causes harm?
Most institutions aren’t ready for these questions. Scientific publishing has no consistent policy for crediting AI. Patent offices are equally unsure how to handle machine-led innovation.
One thing’s clear: we need new frameworks. AI isn’t just an assistant anymore—it’s a creative force that’s rewriting the rules of research.
Algorithmic Bias: Invisible but Invasive
Bias in AI isn’t just a social issue—it’s a scientific one too. Models trained on Western-centric research may miss findings relevant to other populations or climates. In biology, models might underrepresent female data, leading to biased conclusions in drug development.
Even worse, biases are often invisible until damage is done. Because GenAI isn’t transparent about how it reaches conclusions, scientists might trust flawed hypotheses without realizing their origin.
This is where human oversight becomes mission-critical. We can’t let AI guide science blindly—we have to be vigilant co-pilots.
Future Outlook: The Road to Autonomous Research
Looking ahead, autonomous scientific agents may soon become commonplace. Imagine AI that not only proposes hypotheses but designs, runs, and analyzes experiments with minimal human input.
These systems could tackle global-scale problems—from climate modeling to new material synthesis—far faster than we ever could.
But with great power comes the need for great governance. Autonomous research will demand transparent algorithms, explainable AI, and deeply ethical oversight.
Key Takeaways
- AI errors can mislead science, especially when trained on biased or flawed data.
- Ethical and legal frameworks for AI-generated discoveries are still lagging.
- Bias in training data can limit global applicability of AI-led findings.
- The future of science will include autonomous research agents—but only with strong human checks in place.
Expert Opinions, Debates & Controversies in AI-Led Science
As GenAI continues to gain ground in research labs, experts across science, ethics, and AI are weighing in—with excitement, caution, and plenty of debate. Here’s a breakdown of the most compelling perspectives and ongoing controversies.
What Leading Scientists Are Saying
Many top researchers see GenAI as a revolution—not a replacement—for science.
- Demis Hassabis (CEO, DeepMind) has called GenAI a “microscope for ideas,” suggesting it allows humans to probe the unknown at a deeper scale. He credits AI models like AlphaFold with unlocking questions that “biology alone couldn’t solve.”
- Fei-Fei Li (Stanford AI Lab) emphasizes human-centered design. She believes GenAI should augment scientific thinking, not automate it blindly. Her work stresses transparency and the need to align AI tools with diverse human goals.
- Jennifer Doudna (CRISPR co-inventor) has expressed both optimism and concern: “The potential is huge—but so is the risk if we stop asking why an idea works just because a machine suggested it.”
The Ongoing Debate: Should AI Be Credited as a Co-Author?
A growing number of published studies have included AI tools like GPT or AlphaFold as contributors. But can AI be an “author”?
- Supporters argue that when AI contributes a central idea, it deserves formal credit—especially when the idea leads to a major breakthrough.
- Critics push back, stating authorship implies intent, responsibility, and consent—none of which AI possesses.
Some journals, like Nature and Science, now require authors to disclose AI usage. But they stop short of granting authorship to machines.
This isn’t just a semantic issue—it affects academic recognition, patents, and even funding decisions.
Controversy: Can We Trust Black-Box AI in Critical Science?
Many GenAI systems operate without offering transparent reasoning. This has sparked backlash, especially in high-stakes fields like medicine and climate science.
- Some ethicists argue “black-box science” undermines the scientific method, which depends on reproducibility and explainability.
- Others argue that outcomes matter more than process—if AI solves problems faster or better, the “how” is a secondary concern.
Still, demand for explainable AI (XAI) is rising. Tools that visualize model decisions or offer traceable reasoning are gaining favor in sensitive domains.
The Innovation Gap: Rich Labs vs. Underfunded Institutions
Another controversy? Accessibility.
- Elite labs with AI infrastructure are pulling ahead, accelerating discovery at breakneck speed.
- Smaller or underfunded institutions often lack the computing power or expertise to use GenAI tools effectively.
This could create a new kind of digital divide in science—where innovation is centralized, and smaller players struggle to keep pace.
Some open-source initiatives (like Hugging Face’s Science NLP models) aim to close this gap, but the challenge remains significant.
Key Takeaways from the Debate
- GenAI is changing how we think about authorship, ownership, and creativity.
- Ethical concerns around transparency, bias, and trust are still unresolved.
- The future of science may depend on democratizing AI tools—not just building smarter ones.
Final Wrap-Up: The New Frontier of Scientific Discovery
Generative AI is no longer just a tool—it’s becoming a co-creator in the pursuit of knowledge. From proposing hypotheses to designing experiments, it’s reshaping how we approach scientific exploration.
But this evolution demands balance. AI brings speed and scale, but humans bring wisdom and responsibility.
As science moves into this machine-augmented frontier, the most powerful breakthroughs will come from synergy—not surrender. The future belongs to those who know how to collaborate with the unknown.
FAQs
Could GenAI help smaller or underfunded research labs?
Absolutely. One of GenAI’s biggest promises is democratizing scientific research. By automating tasks like literature review, data analysis, and even experimental design, small labs can compete with better-funded institutions.
For instance, a solo researcher could use open-source GenAI tools to scan global data for overlooked treatment options in rare diseases—something that would’ve taken a team months.
It levels the playing field in a big way.
What are the limits of GenAI in discovery today?
Despite the hype, GenAI has major limitations:
- Lack of common sense: It can’t distinguish meaningful patterns from coincidences unless guided carefully.
- No physical intuition: AI doesn’t understand physical constraints or real-world feasibility unless trained with those parameters.
- Opaque reasoning: Most GenAI models are black boxes—you see the output, but not the reasoning behind it.
Can GenAI be biased even if trained on scientific data?
Yes—and this is crucial. Scientific datasets can contain historical biases, especially in fields like medicine, sociology, or psychology. If a GenAI model is trained on unbalanced or exclusionary data, it may reinforce those biases in its hypotheses.
Imagine an AI trained mostly on male-dominant clinical data. Its outputs could overlook symptoms more common in women—leading to incomplete or harmful conclusions.
Bias isn’t just social—it’s structural. And that makes transparency essential.
What’s the difference between a hypothesis and a prediction in AI?
A prediction estimates outcomes based on current inputs. A hypothesis suggests an unproven relationship worth testing.
GenAI can do both. For instance:
- Prediction: This patient is likely to develop Type 2 diabetes.
- Hypothesis: A rare gut microbe may play a causal role in early diabetes onset.
Hypothesis generation is a more creative, open-ended process. And that’s where GenAI is showing unexpected strengths.
How transparent is GenAI about how it generates ideas?
Most GenAI models—especially large language models—are still black boxes. They generate ideas based on complex internal weights and probabilities, but they don’t explain why a certain hypothesis was chosen.
Some newer research is trying to address this with explainable AI (XAI), which highlights which parts of the data led to specific conclusions.
Until then, GenAI’s outputs need careful validation. Trust, but verify.
Could GenAI help reverse-engineer unsolved mysteries?
That’s one of the most exciting prospects. With access to decades of data, GenAI can spot long-overlooked patterns in fields like:
- Archaeology: Linking artifact styles across cultures.
- Theoretical physics: Suggesting relationships between unsolved equations.
- Neuroscience: Connecting brain signals to behavior in new ways.
In some pilot studies, GenAI has even suggested new mathematical models to explain chaotic systems. It’s like giving Sherlock Holmes a supercomputer for a brain.
Resources on GenAI and Scientific Discovery
Here’s a curated list of insightful resources to explore the evolving role of Generative AI in science. Whether you’re a researcher, student, or tech enthusiast, these will deepen your understanding of how machines are shaping the future of discovery.
Articles & Reports
- Nature: Can AI make new scientific discoveries?
A thought-provoking deep dive into how AI is helping shape new hypotheses and redefine what it means to do science. - MIT Technology Review: AI designs drugs in days—not years
Details real-world breakthroughs, including how AI identified a powerful antibiotic never seen before. - Stanford HAI: The Emerging Role of AI in Scientific Research
Covers ethical concerns, research trends, and the role of GenAI in hypothesis formation.
Research Platforms & Tools
- Semantic Scholar’s AI-powered search
A smarter research tool that uses AI to summarize, cluster, and prioritize scientific literature. - IBM Watson for Drug Discovery
An AI solution that helps researchers uncover hidden patterns across biomedical data. - DeepMind’s AlphaFold
Solved the protein folding problem using deep learning, now revolutionizing molecular biology.
Academic Papers
- “The role of AI in science: A framework for ethical collaboration” – PNAS
Discusses models for responsible human-AI partnerships in future scientific inquiry. - “AI in the Scientific Method” – Journal of Artificial Intelligence Research (JAIR)
Explores how generative AI can be incorporated at various stages of scientific methodology.
Learning & Courses
- AI for Science by Caltech & AWS
A free, advanced course introducing AI applications across chemistry, physics, and biology. - Coursera: AI For Everyone by Andrew Ng
Ideal for beginners curious about how AI fits into professional environments—including scientific research.
Journalistic Sources
Isomorphic Labs Secures $600 Million for AI-Driven Drug Discovery
Isomorphic Labs, an AI-focused drug discovery startup spun out of Google’s DeepMind, has raised $600 million in its first external funding round. Led by Thrive Capital and joined by GV and Alphabet, the investment aims to accelerate research and development, expand the team, and advance their AI drug design engine. CEO Demis Hassabis, a Nobel laureate, plans to have AI-designed drugs in clinical trials by the end of the year. The company collaborates with major pharmaceutical firms like Novartis and Eli Lilly. Financial Times
DeepMind Adjusts Research Publication Policies
DeepMind, Google’s AI division, has implemented stricter publication policies to maintain a competitive edge in AI development. The new measures include a more rigorous review process and a six-month embargo on strategic papers, aiming to prioritize commercial applications over academic contributions. This shift has led to frustration among researchers and reflects Google’s broader focus on product development in the AI sector. Financial Times
AI Co-Scientists Accelerate Scientific Breakthroughs
Google Research has introduced an AI co-scientist, a multi-agent system built with Gemini 2.0, designed to assist scientists in generating novel hypotheses and research proposals. This virtual collaborator aims to expedite scientific and biomedical discoveries by providing innovative insights and accelerating the research process. Google
Debate on GenAI’s Role in Scientific Creativity
A recent study examines whether generative AI can make scientific discoveries comparable to those of humans. The findings suggest that while GenAI can generate hypotheses and design experiments, it may lack the intrinsic creativity and intuition that human scientists possess, sparking discussions about the role of AI in research. Nature
AI-Powered Tools Mimic Scientists to Expedite Discoveries
Researchers at Monash University have developed a generative AI tool that simulates the behavior of scientists to support and accelerate the process of scientific discoveries. Published in Nature Machine Intelligence, this tool aims to enhance research efficiency by automating aspects of hypothesis generation and experimental design. Tech Xplore Monash University
AI’s Expanding Influence in Scientific Research
Technologists highlight that AI is driving a revolution in how new knowledge is discovered and utilized. Advances in AI enable scientists to achieve breakthroughs that were previously unattainable, accelerating the pace of scientific discovery and expanding the horizons of research across various disciplines. World Economic Forum
Generative AI Tackles Complex Physics Questions
Researchers have employed generative AI to develop a physics-informed technique for classifying phase transitions in materials or physical systems. This approach is more efficient than existing machine-learning methods and demonstrates AI’s potential in addressing complex questions in physics. MIT News
AI Scientist Framework for Automated Research
A comprehensive framework for fully automated scientific discovery, termed “The AI Scientist,” has been proposed. This system enables large language models to perform research independently, including generating novel ideas, writing code, executing experiments, and communicating findings, marking a significant step toward autonomous scientific research. arXiv