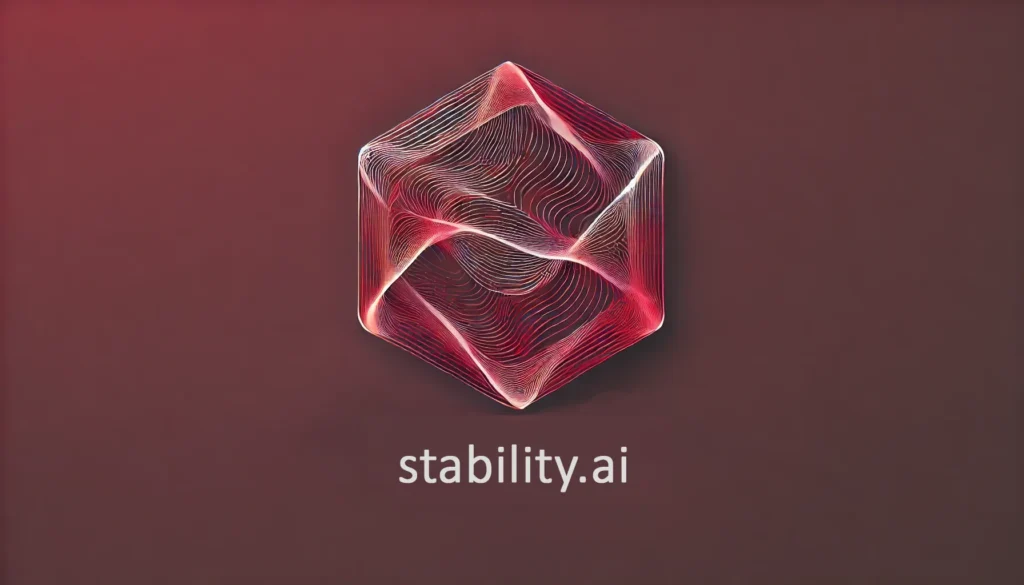
The competition between open-source AI and closed AI has sparked one of the most dynamic debates in the artificial intelligence industry.
Open-source platforms, like Stability AI, are disrupting the traditional AI landscape dominated by tech giants with proprietary, closed systems. Here’s a deep dive into how Stability AI and open-source models are pushing the boundaries and challenging the status quo in AI.
Understanding Open-Source and Closed AI
What Is Open-Source AI?
Open-source AI refers to AI models, tools, and software released under open licenses, allowing anyone to view, modify, and distribute the code. Open-source projects promote collaboration, transparency, and accessibility. They enable developers, researchers, and organizations to freely experiment, which fosters faster innovation and community-driven improvements.
Some examples of open-source AI include:
- Stability AI’s Stable Diffusion: A text-to-image model that anyone can use or modify.
- Hugging Face Transformers: A library of models for NLP, vision, and more.
- OpenAI’s GPT-2: While OpenAI is now moving toward closed models, GPT-2 remains a well-known open-source model.
What Is Closed AI?
Closed AI involves proprietary models developed and maintained by a single organization, with restricted access to the underlying code and architecture. Tech giants like OpenAI, Google DeepMind, and Microsoft follow this approach, aiming to safeguard intellectual property, control quality, and maintain security. Although they release API access to developers, the models themselves remain hidden.
Closed AI companies often cite reasons such as:
- Safety and security: Minimizing misuse by keeping models under close supervision.
- Monetization: Charging for API usage as a revenue model.
- Competitive advantage: Protecting breakthroughs and maintaining market position.
Why Stability AI Embraces Open-Source
Democratizing AI Access
Stability AI’s mission is to make powerful AI tools accessible to everyone. Stable Diffusion, their flagship model, is open-source and available for anyone to download and run on personal hardware. This approach has democratized generative AI, allowing independent developers and small businesses to innovate without paying high fees for proprietary tools.
Stability AI’s emphasis on accessibility contrasts sharply with closed models that require significant investment to access. This open model encourages a community of global contributors, each adding to the model’s robustness and adaptability.
Fostering Transparency and Community Innovation
Transparency is a major benefit of open-source AI. With Stability AI, users have access to complete model details, weights, and configurations. This transparency fosters trust and allows researchers to study and improve model behavior. When issues like bias or accuracy limitations arise, the open-source community can directly collaborate on solutions.
This contrasts with closed AI models, where model decisions and potential biases are often opaque, creating a “black box” effect. By keeping the model open, Stability AI enables researchers to conduct peer reviews and community-driven improvements that drive innovation.
The Advantages of Open-Source AI
Lowering Barriers to Entry
Open-source models like Stable Diffusion lower barriers for startups, academic researchers, and small organizations. Closed models require costly access fees, making them impractical for those with limited resources. Open-source alternatives provide comparable capabilities without high costs, supporting more players in the AI space.
Accelerating Innovation
The collaborative nature of open-source projects accelerates development and innovation. When Stability AI releases a model, anyone can create modifications or extensions, often enhancing functionality far beyond the initial release. Rapid iteration and diverse input help open-source models improve at a faster rate than many closed models.
Greater Ethical Oversight
Open-source AI enables ethical oversight by the community. Users can test for biases, understand how data is used, and identify issues that closed models might hide. This open feedback loop can help models evolve to be more responsible and inclusive over time.
Open-Source AI Unique Challenges:
- Transparency: Open models often expose processes, which can lead to misuse if not managed responsibly.
Closed AI Unique Challenges:
- Proprietary Control: Lack of public scrutiny over algorithms and decision-making processes.
Shared Challenges:
Misuse: Misappropriation of AI technologies occurs across open and closed systems.
Bias: Both systems are susceptible to training data biases.
The Challenges of Open-Source AI
Quality Control and Consistency
One significant challenge for open-source AI is maintaining quality control. Unlike closed AI companies, which strictly manage their releases, open-source projects can experience inconsistent updates and variations. Stability AI must rely on community contributions to identify and fix issues, which may not always be as rigorous or timely as controlled, in-house management.
Security Risks
Open-source AI can potentially expose sensitive functionalities, increasing the risk of misuse. Closed AI companies often argue that restricting access prevents malicious actors from manipulating models for harmful purposes, like deepfake generation or misinformation campaigns. Stability AI must contend with these risks by educating users and setting ethical standards, but it lacks the strict control that closed AI companies possess.
Insights:
Challenges like Quality Control and Community Coordination have moderate urgency and impact, while Scalability is less critical.
Funding has the highest impact and urgency, emphasizing the critical need for sustainable financial support.
Security Risks are urgent but less impactful compared to funding issues.
Limited Monetization Options
Revenue generation is another challenge. Closed AI models can generate income by charging for API access or exclusive features, while open-source models, by nature, are free. Stability AI primarily relies on funding and partnerships, which may not always be stable or sufficient for long-term growth. This limits the resources available for further development compared to the substantial budgets of closed AI players.
How Stability AI Is Competing with Big Players
Developing Scalable, High-Quality Open Models
Stability AI focuses on creating scalable models that run on consumer-grade hardware. Stable Diffusion, for example, is optimized for efficient memory usage, allowing it to run on most laptops and personal computers. This accessibility gives Stability AI a competitive edge by reaching a broader audience than closed models requiring specialized infrastructure.
Cultivating a Thriving Developer Ecosystem
By embracing open-source, Stability AI has cultivated a global network of developers who contribute to the model’s ongoing evolution. Community-created plugins, fine-tuned models, and new applications emerge daily. This ecosystem allows Stability AI to compete with closed AI by leveraging crowd-sourced innovation, which boosts functionality and adoption.
Partnerships with Universities and Research Institutions
Stability AI collaborates with academic and research institutions to drive model improvements and increase reach. By working with universities, Stability AI integrates the latest research directly into their models, often at a fraction of the cost for closed AI companies. These partnerships contribute to advanced research and provide Stability AI with an edge in producing state-of-the-art models.
Building Ethical AI Standards
Stability AI is developing a set of open standards to guide responsible AI use. These standards aim to establish ethical practices for both developers and users, promoting responsible deployment of open-source models. Through these standards, Stability AI addresses some of the ethical concerns that closed AI models cite as reasons for restricted access, positioning itself as a pioneering leader in ethical open-source AI.
Open-Source vs. Closed AI: Examining Key Challenges and Future Potential
As Stability AI continues to push forward with its open-source AI agenda, the challenges and limitations of both open and closed models come into sharper focus. The competition between these approaches is not just a technological battle; it’s a deeper ideological clash over control, innovation, and responsibility. In this section, we’ll explore critical challenges that both open-source and closed AI face and what the future may hold for each.
Transparency: Open-source models excel due to public access to code and processes. Cost: Open-source models are more affordable, with lower licensing fees or free access. Scalability: Closed AI models often leverage robust infrastructure, scoring higher in scalability. Innovation Speed: Open-source models benefit from community-driven innovation. Ethical Oversight: Open-source models show slightly better ethical oversight due to transparency.
Major Challenges for Open-Source AI
Difficulty in Maintaining Long-Term Financial Sustainability
While open-source AI offers access and flexibility, funding is a constant challenge. Open-source projects, such as those by Stability AI, rely heavily on grants, donations, and partnerships to support development. This contrasts sharply with closed AI models that generate substantial revenue by selling access or products.
If open-source AI projects do not secure sustainable financial models, their growth and updates may slow, making it difficult to keep pace with closed systems. Stability AI, for example, has secured funding from venture capital but remains reliant on additional investments to support development and scale. Ensuring ongoing financial health is essential for open-source AI to remain competitive with better-funded closed AI ecosystems.
Funding Type | Open-Source AI | Closed AI |
---|---|---|
Venture Capital | 💼 Limited availability Focus on projects with commercialization potential | 💼 Major source of funding Drives rapid development with ROI-focused goals |
Licensing | 🔑 Minimal reliance Often permissively licensed for public use | 🔑 Key revenue model Heavy reliance on proprietary licensing agreements |
Grants | 🎓 Significant contributor Supported by research institutions and nonprofits | 🎓 Occasionally utilized More common in academia-affiliated closed projects |
Community Donations | ❤️ Primary source Crowdfunded and supported by contributors worldwide | ❤️ Rarely used Typically not applicable for profit-driven projects |
Icons Legend:
- 💼: Venture capital
- 🔑: Licensing
- 🎓: Grants
- ❤️: Community donations
Insights:
- Open-Source AI heavily relies on community support, grants, and limited venture capital.
- Closed AI is dominated by venture capital and licensing revenues, focusing on commercial sustainability.
Balancing Openness with Security and Ethics
One of the most significant criticisms of open-source AI is the potential for misuse. Open models are available for anyone to use, which can sometimes include malicious actors. This lack of control over who accesses the models—and how they’re used—raises ethical questions. Without oversight, open-source models can be applied to areas like misinformation, surveillance, or unauthorized deepfake generation.
Stability AI and other open-source organizations are working to create ethical guidelines and promote responsible use, but without the oversight that closed AI companies have, ensuring adherence to these standards remains challenging. Balancing openness with ethical responsibility will require ongoing community engagement, transparency, and potentially even regulatory support.
Keeping Up with the Rapid Advancements in Closed AI
Closed AI companies, such as OpenAI, Google DeepMind, and Meta, have resources to invest in cutting-edge technology, vast datasets, and high-performance computing. They can rapidly iterate and release state-of-the-art models, often making advancements that open-source initiatives may struggle to replicate at the same pace.
Closed AI can also attract top talent with competitive salaries and substantial resources, which can be a challenge for open-source projects with limited budgets. If open-source models lag significantly behind closed alternatives, the latter may dominate sectors requiring high accuracy, security, or proprietary data processing.
Challenges for Closed AI
Limited Access and High Costs
The closed nature of proprietary AI models means that access is often restricted to paying clients, which excludes smaller developers, startups, and educational institutions. By keeping these models behind paywalls or closed APIs, closed AI companies risk stifling broader innovation and diversity of use cases. This restricted access creates barriers, particularly in developing regions, and prevents AI from reaching its full societal potential.
Stability AI and other open-source efforts present an attractive alternative to those priced out of closed models. If closed AI companies fail to address accessibility concerns, they may cede market share to open-source platforms that offer comparable capabilities at little to no cost.
Transparency and Trust Issues
Closed AI models face criticism for their lack of transparency, often viewed as “black boxes” where users cannot fully understand how models make decisions. This opacity can lead to trust issues among users, particularly in industries like healthcare, finance, and public policy where accountability is crucial. Unlike open-source models, which are scrutinized and improved by a wide array of contributors, closed AI models are limited to in-house review, which may be perceived as less objective.
Closed AI companies are beginning to address this by incorporating transparency features, such as explainable AI tools, but these efforts are still in the early stages and lack the openness of community-led initiatives like Stability AI. If transparency concerns are not effectively managed, trust in closed AI systems may erode, making open-source AI more appealing to those prioritizing transparency.
Vulnerability to Regulatory Constraints
As governments develop regulations for AI, proprietary models could face strict oversight in areas such as privacy, bias, and accountability. Closed AI companies, which often operate with limited public scrutiny, are vulnerable to increased regulation because regulators may view them as high-risk for issues like bias or misuse.
Open-source AI, on the other hand, has an advantage in regulatory environments focused on transparency and accessibility. Stability AI’s community-driven approach aligns well with potential regulatory demands for openness, ethics, and inclusivity. If regulations favor transparent, community-driven models, closed AI companies may face operational challenges that could limit their ability to scale or access certain markets.
The Path Forward: The Future of Open vs. Closed AI
Hybrid Models: A Blended Approach to AI Development
As open-source and closed AI evolve, a hybrid model—incorporating the best of both approaches—may emerge. Hybrid AI could involve open-source foundational models that are extended or customized with closed, proprietary layers for specific applications. This would allow companies to retain control over high-stakes applications while benefiting from the transparency, community insights, and innovation inherent in open-source foundations.
Some companies, including Google, are already experimenting with partially open models, where base-level frameworks are available to the public but customized versions are kept proprietary. This trend could foster more collaboration across the industry while retaining flexibility for organizations to protect proprietary innovations.
Regulatory Influence on Open and Closed AI
As AI technology advances, government regulations will likely become more prominent. Open-source AI, with its emphasis on transparency, may align better with regulatory frameworks that prioritize accountability, user rights, and ethical standards. Stability AI and similar organizations could be well-positioned to adapt to emerging regulations that demand greater transparency and oversight in AI applications.
Conversely, closed AI companies may face regulatory challenges unless they address transparency, fairness, and accessibility concerns. Governments may favor AI solutions that promote social good and inclusivity, potentially giving open-source projects a competitive edge in regulated industries, such as finance, healthcare, and public services.
The Role of AI Ethics and Community Governance
Ethical concerns around AI will continue to shape the industry, especially as AI becomes more integrated into everyday life. Open-source projects like Stability AI, which prioritize community governance, may drive ethical standards across the industry. With a collective approach to governance, open-source AI communities can better self-regulate and address bias, keeping pace with evolving ethical standards.
Closed AI companies may also adopt some level of community governance or advisory councils, especially as ethical concerns and user trust grow. An open-dialogue approach, involving diverse stakeholders, could bridge the ethical gap between open and closed models, providing a framework for responsible AI that benefits both communities and commercial interests.
Conclusion: A New Landscape for AI
The rise of Stability AI and the open-source model has challenged the traditional closed AI landscape, introducing a more inclusive, accessible approach that empowers developers worldwide. However, both models face significant challenges, from funding and security in open-source to transparency and regulatory pressures in closed AI. The competition between these approaches is not likely to end in a single “winner”; instead, it will reshape the AI ecosystem into a more diverse, multi-dimensional landscape where both open and closed models coexist and cater to different needs.
The future of AI is likely to be a blend of collaborative open-source innovation and proprietary, application-specific enhancements. By continuing to address their respective challenges, Stability AI and other open-source platforms can foster a robust and inclusive AI ecosystem, pushing tech giants to prioritize transparency, accessibility, and ethical AI practices. As these shifts unfold, we may witness a new era of AI development, one that balances commercial interests with societal good, ensuring that AI’s benefits are truly available to all.
FAQs
What are the security risks associated with open-source AI?
Open-source AI can be accessed and modified by anyone, which raises the risk of misuse, such as generating deepfakes or malicious bots. Stability AI and other open-source platforms are working to establish ethical guidelines and responsible use policies, though enforcing these across a global community can be challenging.
How does closed AI benefit from keeping models proprietary?
Closed AI companies benefit by controlling access to their models, which enables them to manage quality, security, and monetization. Restricting model access allows these companies to offer specialized services and protect their intellectual property while charging for API or subscription-based usage.
Can open-source and closed AI coexist in the industry?
Yes, open-source and closed AI can coexist and complement each other. Many companies use open-source frameworks for foundational tools while maintaining proprietary layers for specific applications. This hybrid approach combines the benefits of community-driven innovation with controlled application for specialized tasks.
Is Stability AI at a disadvantage compared to large closed AI companies?
While Stability AI operates on a different financial model, relying on community support and partnerships rather than large corporate budgets, its open-source approach offers advantages in agility, transparency, and community-driven innovation. Stability AI’s accessibility allows it to compete in ways that closed AI companies may find challenging.
How do open-source AI projects stay financially sustainable?
Open-source AI projects like Stability AI often rely on venture capital, donations, and partnerships. Some open-source projects offer premium services or consulting as revenue streams. While this model can be less predictable than closed AI’s revenue from proprietary services, it allows for widespread adoption and community support.
Could government regulations impact open-source AI differently from closed AI?
Yes, open-source AI may be favored in regulatory environments that prioritize transparency and ethical use. Stability AI’s commitment to openness aligns with many regulatory expectations for accountability and bias prevention. Closed AI companies might face more stringent oversight if their proprietary nature limits public or regulatory access to model workings.
How does open-source AI democratize access to technology?
Open-source AI removes the barriers of cost and exclusivity by making powerful models freely available. This democratization allows startups, independent developers, researchers, and organizations with limited resources to access and leverage AI, encouraging innovation across industries without the high fees associated with closed AI.
Can open-source AI models be integrated into commercial products?
Yes, open-source AI models can be used in commercial applications, provided that the licensing terms allow for it. Many open-source licenses, such as Apache or MIT, permit commercial use, though some require attribution or restrict certain modifications. Stability AI’s models are designed with broad applications in mind, including commercial integration.
How does Stability AI handle model updates and improvements?
Stability AI’s open-source nature means that model improvements are often community-driven. The community contributes to enhancements, bug fixes, and new features, creating a collaborative ecosystem where updates happen rapidly. Stability AI can release official updates, but community input speeds up model evolution and adaptability.
Why do some companies prefer closed AI for their models?
Closed AI provides companies with complete control over model access, use, and revenue generation. Closed models are easier to monetize through licensing and subscription services, which makes them appealing for companies looking to protect proprietary technology and maintain a competitive edge in AI services.
Are open-source AI models more likely to be biased?
Bias in AI models can occur in both open and closed systems, often stemming from the data used to train the models. Open-source models can actually benefit from community scrutiny, as a larger pool of users can help identify and address biases more effectively than closed models, where the data and model training methods may remain hidden.
How does Stability AI address ethical concerns?
Stability AI works to promote ethical use through transparency and guidelines on responsible AI deployment. By making models open-source, they allow researchers and users to analyze model behavior and biases, encouraging ethical improvements. Stability AI also collaborates with industry groups to establish standards for responsible AI use.
What role does community play in open-source AI development?
Community plays a central role by contributing code, suggesting features, testing for biases, and developing third-party integrations. Stability AI’s community-driven approach enables faster improvements and allows the model to evolve to meet diverse needs. This decentralized development can lead to more adaptable and inclusive AI tools.
Do open-source AI models face challenges in scalability?
Scaling open-source models can be challenging, especially for complex tasks that require extensive computing resources. While Stability AI designs its models to run on consumer-grade hardware, large-scale deployment still requires significant resources. Some open-source projects partner with cloud providers or implement funding models to offer scalable solutions.
Is open-source AI safer than closed AI?
Open-source AI offers transparency, which can enhance safety by allowing the community to review and understand the model’s inner workings. However, open access also introduces risks of misuse. Stability AI emphasizes responsible use and provides guidelines, but ultimately, open-source AI requires users to exercise ethical responsibility.
How does Stability AI stay competitive with industry giants?
Stability AI leverages its open-source model to create a community of global contributors who rapidly innovate and improve the model, providing a competitive edge through crowd-sourced development. This allows Stability AI to keep pace with larger, closed AI organizations by offering accessible, high-performance models without the barriers associated with proprietary platforms.
Will open-source AI lead to faster innovation?
Open-source AI can accelerate innovation by allowing anyone to contribute improvements and adapt models for diverse applications. With Stability AI’s open approach, new features, extensions, and fixes often emerge quickly from the community, enabling faster and broader development than closed models limited to in-house teams.
What is the future outlook for open-source vs. closed AI?
As demand for transparency and ethical AI grows, open-source AI is likely to play a more significant role, especially in sectors where accountability is critical. Closed AI will continue to dominate high-stakes applications requiring controlled access, but the industry may evolve towards hybrid models that incorporate open-source foundations with proprietary enhancements. Stability AI and similar platforms are setting the stage for a future where open and closed AI can coexist and drive the industry forward together.
Resources
Open-Source Platforms and Libraries
- Hugging Face
- Description: A hub for open-source models and datasets focused on NLP, vision, and other AI domains. Hugging Face offers a community-driven platform for sharing and improving models.
- Link: Hugging Face
- Best For: Finding and contributing to open-source AI models for a wide range of applications.
- GitHub – Stability AI’s Stable Diffusion
- Description: Stability AI’s popular text-to-image model repository on GitHub, where the community can download, experiment with, and contribute to the model.
- Link: Stable Diffusion on GitHub
- Best For: Accessing the source code, experimenting, and engaging with Stability AI’s open-source community.
- TensorFlow and PyTorch
- Description: Two of the most widely-used open-source frameworks for machine learning and deep learning, supported by extensive communities.
- Link: TensorFlow and PyTorch
- Best For: Building and deploying machine learning models, including applications using Stability AI’s technology.
- OpenAI Gym
- Description: Although OpenAI is now more closed, OpenAI Gym remains a valuable open-source toolkit for developing reinforcement learning algorithms.
- Link: OpenAI Gym
- Best For: Learning reinforcement learning in an open-source environment, providing context for how Stability AI’s openness compares.
Community Forums and Discussion Groups
- Stability AI Discord Server
- Description: Stability AI’s official Discord server, where developers, researchers, and enthusiasts discuss developments, improvements, and best practices for open-source AI models.
- Link: Stability AI Discord
- Best For: Real-time interaction with Stability AI’s community and developers, discussing project updates and collaborations.
- r/MachineLearning on Reddit
- Description: A subreddit dedicated to discussing advancements in machine learning, including open-source vs. closed models and trends in AI development.
- Link: r/MachineLearning
- Best For: Staying updated on the latest in AI research, including perspectives on open-source projects.
- AI Alignment Forum
- Description: A forum focused on the ethical, technical, and safety aspects of AI, with discussions on open-source AI’s role in promoting safe and transparent development.
- Link: AI Alignment Forum
- Best For: Insights into the ethical and safety considerations of AI, useful for understanding the responsibility aspect of Stability AI’s mission.
- Kaggle
- Description: A data science and machine learning community that provides datasets, competitions, and forums, often focused on open-source projects and collaborative problem-solving.
- Link: Kaggle
- Best For: Practicing model development, finding open-source datasets, and participating in AI challenges.
- Quantitative Finance Stack Exchange
- Description: A Q&A site where finance and AI enthusiasts discuss the use of machine learning, including open-source tools in finance and trading applications.
- Link: Quantitative Finance Stack Exchange
- Best For: Learning about applications of open-source AI models in finance, where transparency is particularly valued.