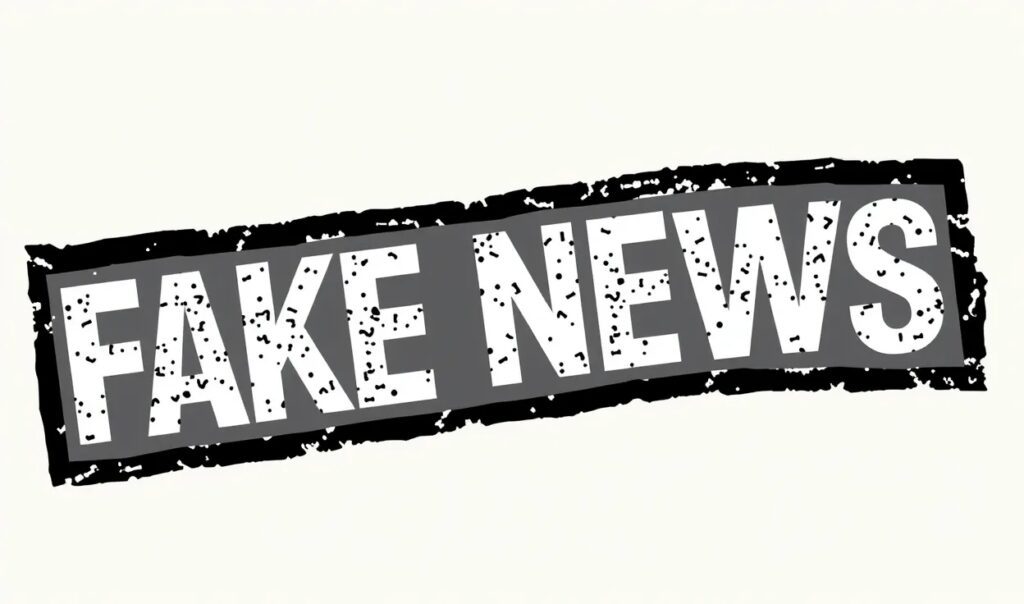
What Is Text Mining and Why Is It Crucial Today?
Decoding Text Mining in Simple Terms
Text mining is a method of analyzing vast amounts of unstructured text data to extract meaningful insights. Think of it as a digital detective: uncovering patterns, trends, and hidden truths in texts like social media posts, articles, or emails.
This process involves natural language processing (NLP), machine learning, and algorithms that sift through data. As text dominates the digital world, text mining becomes crucial for making sense of the overwhelming flow of information.
The Rise of Misinformation in the Digital Age
With the internet, information spreads at breakneck speed. Unfortunately, fake news and misinformation spread just as fast, often disguised as credible facts. Fake news thrives on our biases and emotions, creating a dangerous ripple effect of confusion and mistrust.
This is where text mining technologies step in. They help detect misleading content, enabling organizations to fight back against the tide of disinformation.
How Text Mining Helps Spot Fake News
Identifying Patterns in Language
Fake news often uses specific linguistic traits: sensational headlines, emotionally charged words, and exaggerated claims. Text mining algorithms are trained to recognize these cues, flagging potential misinformation for further review.
For example, tools like LIWC (Linguistic Inquiry and Word Count) analyze text for emotional tone and deceptive markers, helping differentiate factual news from dubious claims.
Evaluating Source Credibility
Not all sources are created equal. Text mining systems evaluate the reputation and reliability of news sources by analyzing their history, content consistency, and fact-checking credibility.
Cross-referencing information from reputable sources like FactCheck.org or Snopes ensures data accuracy and helps distinguish truth from fiction.
Sentiment Analysis: A Key Weapon Against Fake News
What Is Sentiment Analysis?
Sentiment analysis is a powerful feature of text mining. It identifies the emotional tone behind text, categorizing it as positive, neutral, or negative. Fake news often thrives on negative sentiment to provoke outrage or fear, making it a prime target for detection.
Real-World Applications of Sentiment Analysis
Governments and organizations use sentiment analysis to track public reactions to crises and detect fake viral content. For example:
- During elections, this helps detect misinformation campaigns targeting specific groups.
- In health crises, it identifies false medical claims, safeguarding public safety.
By breaking down emotional patterns, sentiment analysis exposes manipulative narratives.
Challenges in Text Mining for Fake News Detection
Evolving Misinformation Tactics
Fake news creators continuously adapt their strategies, making detection more complex. They use advanced AI tools, bot accounts, and even human influencers to make their content appear legitimate.
Text mining tools must evolve just as quickly to stay ahead. This involves integrating deep learning models that improve accuracy over time.
Language Diversity and Regional Variations
Fake news isnโt limited to one language. From English to local dialects, misinformation finds its way into global audiences. Developing multilingual NLP models that understand cultural context is a major hurdle in combating this problem.
The Role of AI and Machine Learning in Fake News Detection
How AI Powers Text Mining
AI enhances text mining by enabling it to learn and adapt from data. Machine learning models process billions of data points, improving their ability to detect fake news over time. These systems donโt just look for patternsโthey predict and adapt to new forms of misinformation.
Key AI-Powered Tools in Use Today
- Fake News Challenge: A competition fostering the development of fake news detection tools.
- Hoaxy: Tracks the spread of false information across social media platforms.
AI-driven text mining ensures constant vigilance against evolving threats.
The Social Media Factor: Fake News Amplified
How Social Media Fuels Misinformation
Social media platforms are fertile ground for fake news due to their speed and scale. Algorithms prioritize engaging content, often promoting sensational or emotionally charged posts over verified news. This “clickbait culture” amplifies the reach of false narratives.
Moreover, echo chambers reinforce misinformation. People are often exposed to ideas aligning with their beliefs, making it easier for fake news to gain traction within specific communities.
Detecting Fake News on Social Media with Text Mining
Text mining on social platforms focuses on identifying patterns like:
- Virality markers (high shares, quick spread).
- Hashtag analysis to trace misinformation campaigns.
- Bot activity detection to pinpoint non-human accounts driving fake narratives.
For instance, tools like Botometer analyze Twitter accounts to flag potential bot behavior.
Fact-Checking at Scale: Automation vs. Manual Efforts
Automated Fact-Checking with Text Mining
Text mining enables automated fact-checking, where algorithms verify claims against trusted databases in real time. This method is faster and scalable, especially useful for platforms handling millions of posts daily.
Examples of automated fact-checking include:
- ClaimBuster, which identifies factual statements and checks their validity.
- Google Fact Check Explorer, allowing users to cross-reference suspicious claims.
Why Manual Fact-Checking Still Matters
Despite advances, human intervention is essential. Automated tools sometimes fail to understand nuanced language, sarcasm, or regional context. Expert fact-checkers provide the cultural sensitivity and judgment necessary to fill these gaps.
Combining human expertise with automation ensures a robust defense against misinformation.
Ethical Concerns in Text Mining for Fake News
Balancing Detection with Privacy
One major concern is balancing fake news detection with user privacy. Text mining often involves analyzing large datasets from public and private communications, raising questions about data misuse.
Governments and organizations must establish clear guidelines to ensure that combating fake news doesnโt compromise user rights.
Bias in AI Models
AI tools can inherit biases from training data, leading to unfair targeting or false positives. For instance, minority groups might be disproportionately flagged based on stereotypes embedded in the dataset.
Addressing bias requires diverse data sources and ongoing refinement of algorithms to reduce errors and ensure fairness.
Global Collaboration: A Unified Fight Against Misinformation
Why Global Efforts Are Necessary
Fake news knows no borders. It affects elections, public health, and even international relations. Countries must collaborate to share resources, technology, and expertise in combating misinformation.
Initiatives Leading the Charge
- The International Fact-Checking Network (IFCN) promotes fact-checking globally.
- UNESCOโs Media and Information Literacy programs educate citizens on identifying and rejecting fake news.
These collaborations ensure that the fight against misinformation is both localized and globally impactful.
What the Future Holds for Text Mining and Fake News Detection
Emerging Technologies in Fake News Prevention
The future of text mining lies in neural networks and transformer models like GPT. These technologies promise enhanced understanding of context, sarcasm, and evolving misinformation patterns.
Additionally, blockchain technology could offer verification trails for news, ensuring transparency and trustworthiness.
Empowering Individuals to Spot Fake News
While technology plays a crucial role, educating individuals is equally important. Teaching media literacy and critical thinking will empower people to identify misleading content, reducing their susceptibility to misinformation.
By combining advanced tools with informed individuals, we can create a more resilient digital landscape.
Real-World Success Stories in Fake News Detection
How Organizations Are Winning the Fight
Several organizations have implemented text mining solutions to combat fake news effectively. Success stories provide valuable insights into how this technology works in practice:
- Facebookโs AI Systems: Using text mining and machine learning, Facebook reduces the spread of misinformation by flagging suspect content and reducing its visibility in news feeds. Collaborations with independent fact-checkers enhance their detection capabilities.
- BBCโs Beyond Fake News Initiative: This initiative combines text analysis and public education to address misinformation in multiple languages, ensuring impact across diverse demographics.
These examples highlight the practical impact of combining AI-driven detection tools with partnerships and education.
Public-Private Partnerships in Action
Public and private sectors are joining forces to tackle fake news at scale. Initiatives like EUvsDisinfo, run by the European Union, deploy text mining tools to expose disinformation campaigns, particularly those targeting democratic processes.
How Text Mining Transforms Fake News Reporting
Journalistic Applications of Text Mining
For journalists, text mining acts as a powerful ally. It helps analyze vast volumes of data, uncovering hidden connections in complex misinformation campaigns. Investigative teams can identify trends, track sources, and debunk fake news faster than ever.
For instance, The Washington Post leverages AI and text mining tools to monitor misinformation online, improving their coverage of critical stories.
Enhancing Newsroom Productivity
By automating repetitive tasks like fact-checking and keyword analysis, text mining frees up journalists to focus on in-depth reporting. This improves the overall quality of news while combating disinformation.
Challenges Ahead: Staying Ahead of the Curve
The Arms Race Against AI-Generated Fake News
As AI advances, so do the tools used by fake news creators, such as deepfakes and realistic AI-generated text. These technologies mimic legitimate content so convincingly that even sophisticated detection systems can struggle.
To counteract this, researchers are developing adversarial AI models that simulate fake news to train detection systems.
Building Trust in Technology
One lingering challenge is public skepticism about using AI and text mining to filter content. Transparency about how algorithms work and ensuring bias-free operations are critical to building trust in these technologies.
The Final Word: A Collaborative Approach to Fighting Fake News
The battle against misinformation is far from over. Text mining, combined with AI-powered tools, is a key weapon in detecting and stopping fake news in its tracks. But technology alone cannot solve the problem. Collaboration among governments, tech companies, media organizations, and citizens is essential.
By pairing advanced tools with a culture of critical thinking and media literacy, we can reduce the impact of fake news and create a more informed digital society.
FAQs
How do journalists use text mining to combat misinformation?
Journalists use text mining to identify false claims, trace their origins, and debunk misinformation efficiently. For instance, newsrooms like Reuters use AI-powered tools to monitor trending topics and flag suspicious narratives for further investigation.
By automating tasks like data collection and sentiment analysis, text mining empowers reporters to focus on in-depth, factual reporting.
Is it possible to completely eliminate fake news with text mining?
While text mining significantly reduces the spread of fake news, itโs unlikely to eliminate it entirely. Misinformation evolves constantly, with creators employing new tactics like AI-generated content.
Combating fake news requires a multi-pronged approach, combining advanced detection tools, human fact-checking, and public education to promote critical thinking and digital literacy.
What are some examples of text mining tools for fake news detection?
Popular tools include:
- Factmata: An AI tool that analyzes text for misinformation and biased language.
- AdVerif.ai: Identifies fake news in advertisements and online articles.
- Fakenews.ai: Specializes in detecting hoaxes and disinformation campaigns.
These tools are used by companies, governments, and individuals to counter misinformation more effectively.
How do echo chambers amplify fake news, and can text mining address this?
Echo chambers are online environments where users are exposed only to content that reinforces their beliefs, often amplifying fake news. Algorithms on social media feed users similar content based on their past interactions, creating confirmation bias loops.
Text mining can help identify echo chambers by analyzing interaction patterns, shared links, and group discussions. For instance, researchers used network analysis tools to study how fake news about COVID-19 vaccines spread within Facebook groups, enabling interventions to disrupt the flow.
What challenges do text mining tools face when detecting fake news?
Text mining tools face challenges like:
- Sarcasm detection: Fake news can use subtle irony or humor, making it hard to classify.
- Misinformation in images: Memes and infographics often contain misleading information not easily detectable by text-based systems.
- Rapidly evolving tactics: Fake news creators use AI to generate more convincing content, like deepfake videos or fabricated text.
For example, detecting a sarcastic tweet like โSure, the Earth is totally flat. Just like a pancake!โ requires advanced NLP models trained on nuanced language.
How can individuals use text mining tools to verify information?
Some text mining tools are user-friendly and accessible to the public. Individuals can input suspicious content into platforms like:
- Fact Check Explorer by Google: Allows users to verify claims with a simple search.
- Hoaxy: Tracks the spread of misinformation on social media, showing how and where it originated.
By leveraging these tools, individuals can independently verify content and avoid spreading fake news.
Can text mining detect AI-generated fake news or deepfakes?
Detecting AI-generated fake news is a growing challenge. Modern tools like OpenAIโs GPT models can create realistic fake articles, making them harder to identify. Text mining algorithms are now incorporating deep learning models that analyze writing style, sentence structure, and content consistency to detect AI-generated content.
For example, researchers at MIT developed a system that identifies subtle patterns in AI-generated text, like predictable sentence structures or overuse of certain phrases, to flag synthetic content.
What can governments do to support text mining efforts against fake news?
Governments can invest in research, establish regulatory frameworks, and promote international cooperation to strengthen text mining tools. For example:
- Funding AI development: Programs like the DARPA Media Forensics initiative develop technologies to detect and counter fake news.
- Legislation against misinformation: Laws requiring platforms to report fake news detection methods ensure transparency.
- Public education campaigns: Governments can teach citizens to critically assess information and spot disinformation.
By combining technology with policy and education, governments can create a more informed and resilient public.
How can schools incorporate fake news education alongside text mining?
Schools can teach students how to use text mining tools while fostering critical thinking skills. For instance:
- Assignments where students use tools like Google Fact Check Explorer to verify claims in trending news.
- Workshops on understanding how language patterns and emotional appeals are used in fake news.
Educating young people equips them to navigate a digital world filled with misinformation, ensuring they become responsible digital citizens.
Resources
Online Tools for Fact-Checking and Misinformation Detection
- Google Fact Check Explorer: Search claims to find reliable fact-checking sources.
- Hoaxy: Visualizes how misinformation spreads on social media.
- Botometer: Detects bot accounts driving disinformation campaigns on Twitter.
- Snopes: A trusted resource for debunking fake news and urban legends.
Research Papers and Studies
- “The Science of Fake News” (Science, 2018): Explores the psychological and social factors that enable misinformation to thrive.
- “Automatic Detection of Fake News Using NLP” (Journal of Machine Learning, 2020): Discusses text mining techniques to detect fake news.
- “Echo Chambers on Social Media” (PNAS, 2016): Examines the role of algorithms in creating echo chambers that spread misinformation.
Organizations and Initiatives
- International Fact-Checking Network (IFCN): A global alliance of fact-checkers working to combat misinformation.
- EUvsDisinfo: Tracks and debunks disinformation campaigns targeting European nations.
- The Trust Project: Promotes transparency in journalism to restore trust and reduce misinformation.
Podcasts and Videos
- The Data Skeptic Podcast: Episodes on fake news detection and the role of AI in combating misinformation.
- PBSโs The Future of Fake News: A short documentary on the evolving landscape of misinformation.
- Voxโs Explained: Fake News: A video series breaking down how fake news impacts society and how it can be addressed.
Government and Policy Reports
- “Countering Disinformation: Lessons from the EU” (2020): Details the European Unionโs strategies for addressing fake news with technology and policy.
- “The Role of AI in Misinformation” (UNESCO, 2021): Analyzes how artificial intelligence combats fake news globally.
- “Combating Misinformation in the U.S.” (FCC, 2019): Discusses legislative approaches to regulating fake news on social media.